“Customers don’t care about your solution, they care about their problem!“
Background: Jobs to Be Done (JTBD) prioritizes user needs and wants instead of focusing on IBM’s offering or solution. It focuses more on the desired outcome of a job rather than on the technology. Having a clear understanding of the jobs users need to do to accomplish their goals creates an environment where innovation within our offerings surface with more ease and clarity.
Objective: This research was conducted to understand the main jobs and job steps for key roles in the data and AI lifecycle - Data Engineer, Data Steward, Data Scientist, and Machine Learning Engineer, as well as to determine the top opportunities and table stakes.
Role & Method: I led Data Engineer squad, a team of 4 researchers total. Our process involved secondary research, stakeholder interviews (n=7), primary research (n=17), and an outcome-driven innovation survey (n=161).
Business Outcomes: For the Data Engineer role, we distilled the findings into a main job (n=1), job steps (n=28), desired outcome statements (n=68), social jobs (n=28), emotional jobs (n=28), and challenges (n=28). Additionally, we identified 3 crucial opportunity areas for Data Engineers in the data and AI lifecycle:
Minimize the risk of inconsistency and mistakes of manual processing.
Maximize the clarity of requirements for the job of migrating and transforming data.
Maximize the efficiency of migrating and processing data.
Next Steps: Researchers will facilitate workshops with the relevant offering stakeholders to discuss:
The as-is states of each outcome
Any to-be plans for each outcome
Priority of each outcome
Secondary Research & Stakeholder Interviews
We started the Jobs to Be Done (JTBD) study by educating ourselves on the JTBD method. Each researcher read The Jobs to Be Done Playbook by Jim Kalbach. We took notes, then made our own plan for moving forward.
To begin learning about the role of a Data Engineer, my team collected secondary research from scholarly articles, news articles, blog posts, LinkedIn profiles, and job postings. I curated and facilitated a kick-off workshop organizing, synthesizing and making sense of all of the research we collected.
We followed up the secondary research with stakeholder interviews. This group was comprised of designers, product managers, and developers. The goal was to collect all of the questions and assumptions our stakeholders had regarding the Data Engineer role before beginning the interviews in order to ensure we addressed all of the questions and assumptions from the team by the end of our study.
The Jobs to Be Done Playbook by Jim Kalbach.
Conducted 7 interviews across Design, Product Managers, and Development
Drafted main job and outcome statements
Identified knowledge gaps
Read Jobs to Be Done by Jim Kalbach
Read MD & I market analyses
Reviewed user jobs from past research
Workshop to synthesize information
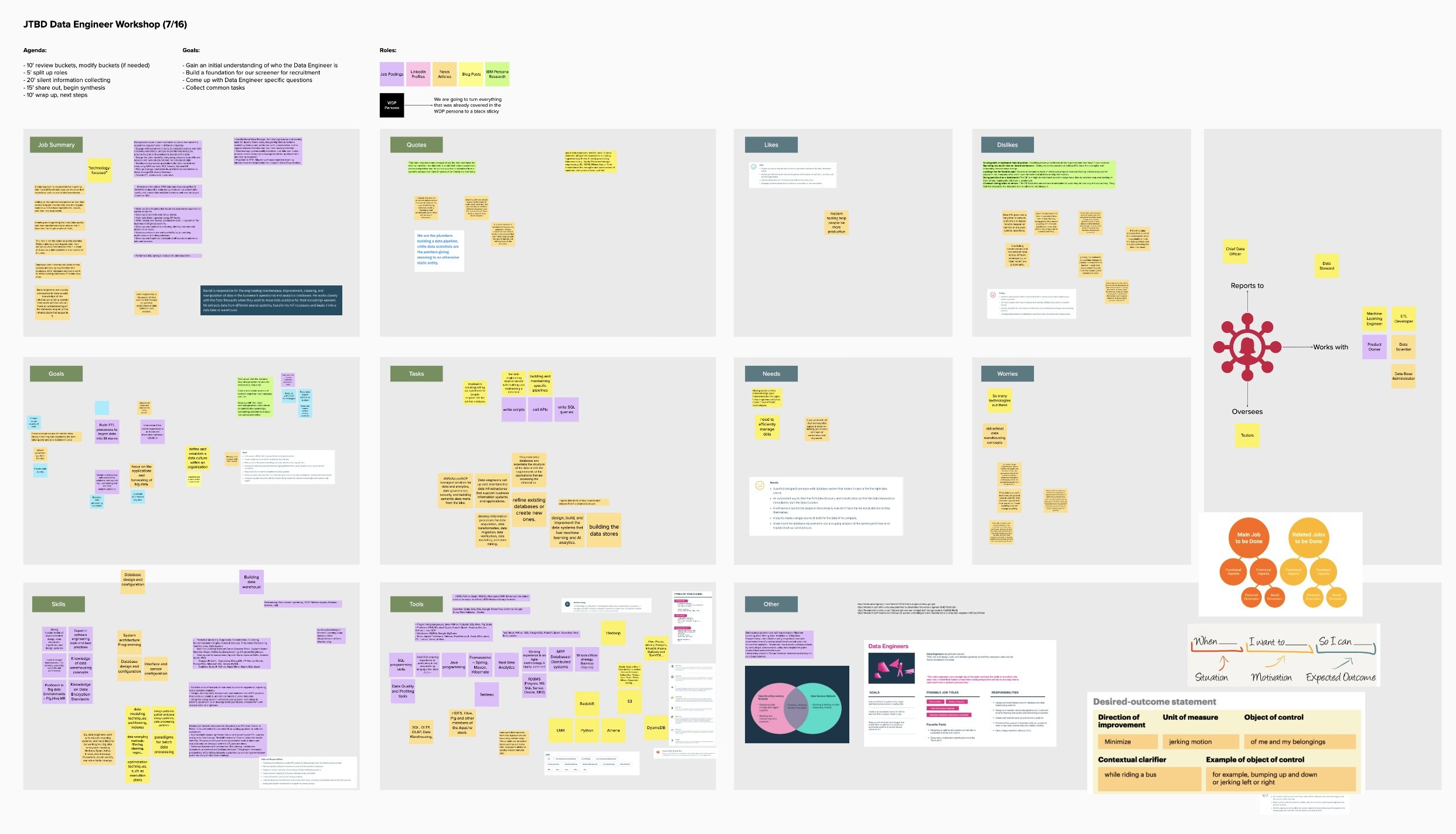
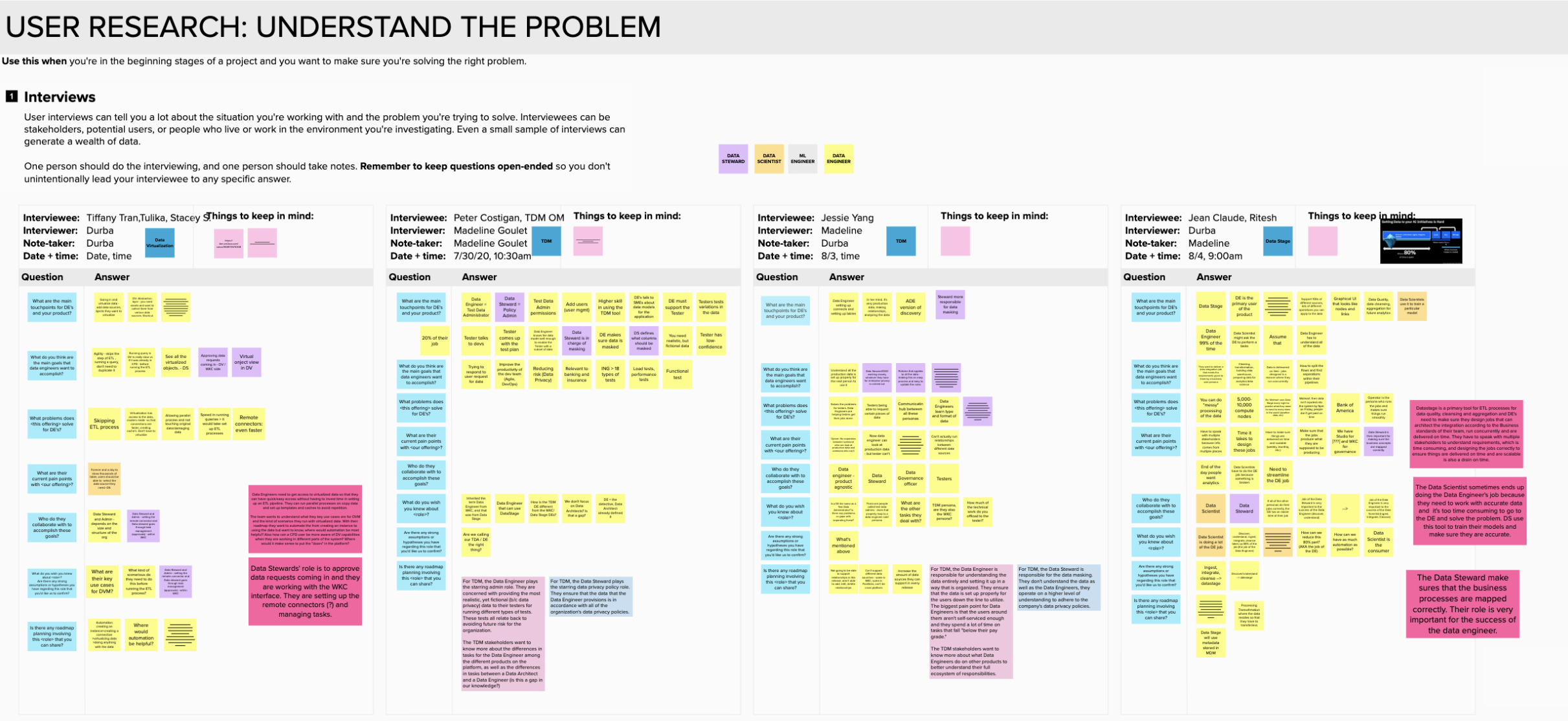
Jobs to Be Done Interviews
For the interview phase, we first put together an interview guide and a note-taking framework that lended itself well to synthesis. We ran a pilot interview to test both artifacts, and iterated on the original frameworks.
The next step was recruitment. We recruited Data Engineers from diverse geographies, industries, and experience levels. Participants came from Respondent, User Interviews, LinkedIn and IBM’s sponsor user program.
Using the synthesis framework, we took notes for each individual interview. After all interviews were completed, We synthesized all of the charts together to create one comprehensive, all-inclusive job map for the role of the Data Engineer. We arrived at one main job, 28 job steps, 68 desired outcome statements, 28 social jobs, 28 emotional jobs, and 28 challenges.

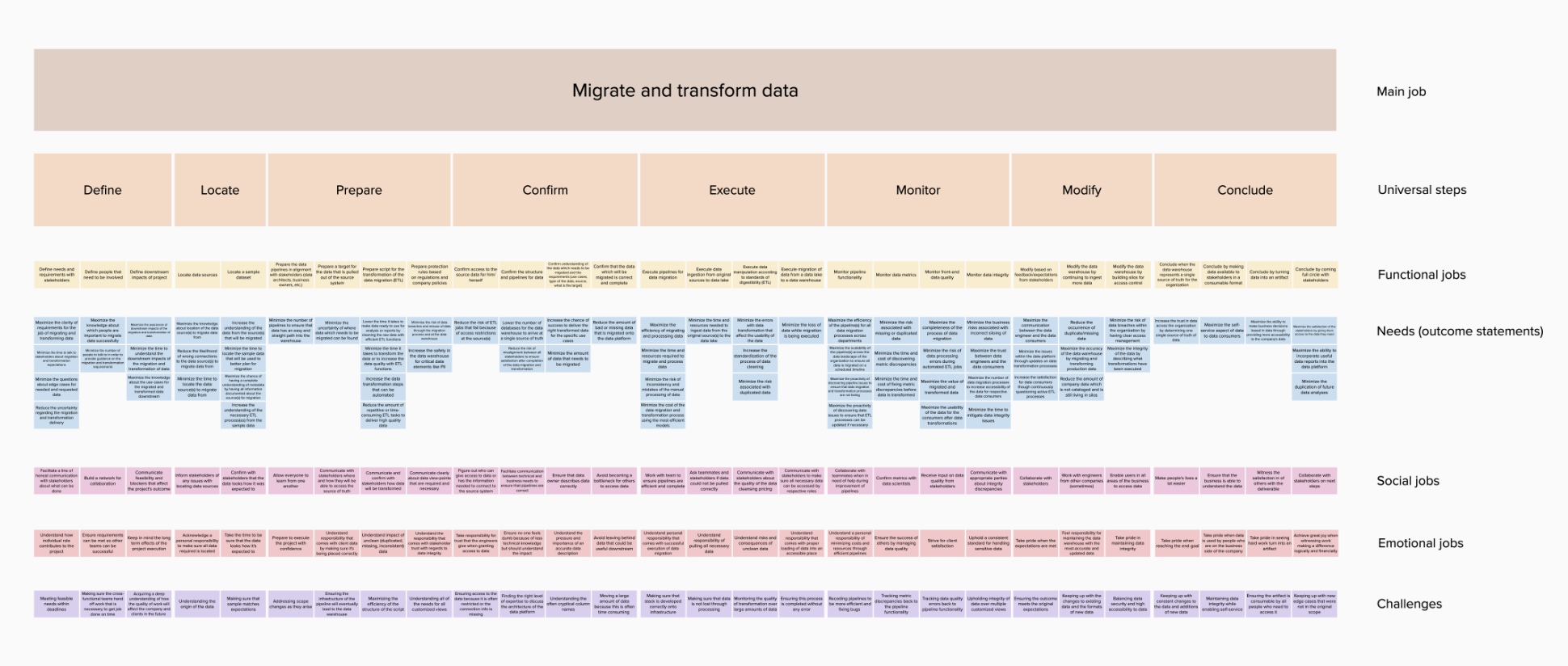
Conducted 17 interviews with prospective and current customers sourced via Respondent, UserInterviews, LinkedIn and UX program participants
Finalized the main job and the 68 outcome statements
Finalized the job map for the Data Engineer role
Outcome-Driven Innovation (ODI) Survey
To identify the opportunity areas for each of the roles, we combined our research from the JTBD interviews across all four squads (Data Engineer, Data Scientist, Data Steward, ML Engineer).
The outcome statements drove the ODI survey. Each outcome statement was rated according to:
How important it was to the participant
How satisfied they were with how it is currently done
All 161 completed survey responses were analyzed and each outcome statement was ranked and given an opportunity score.
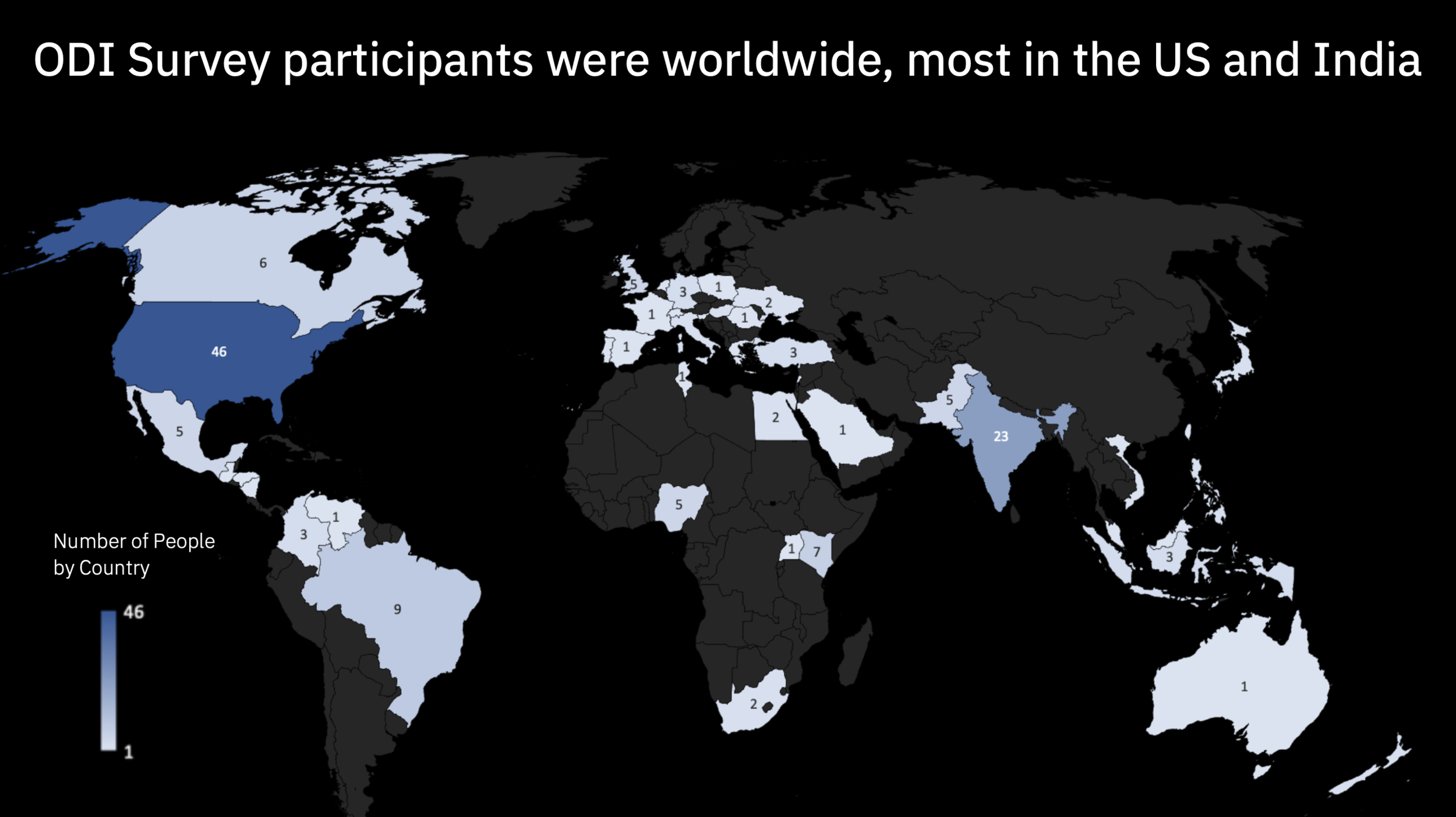
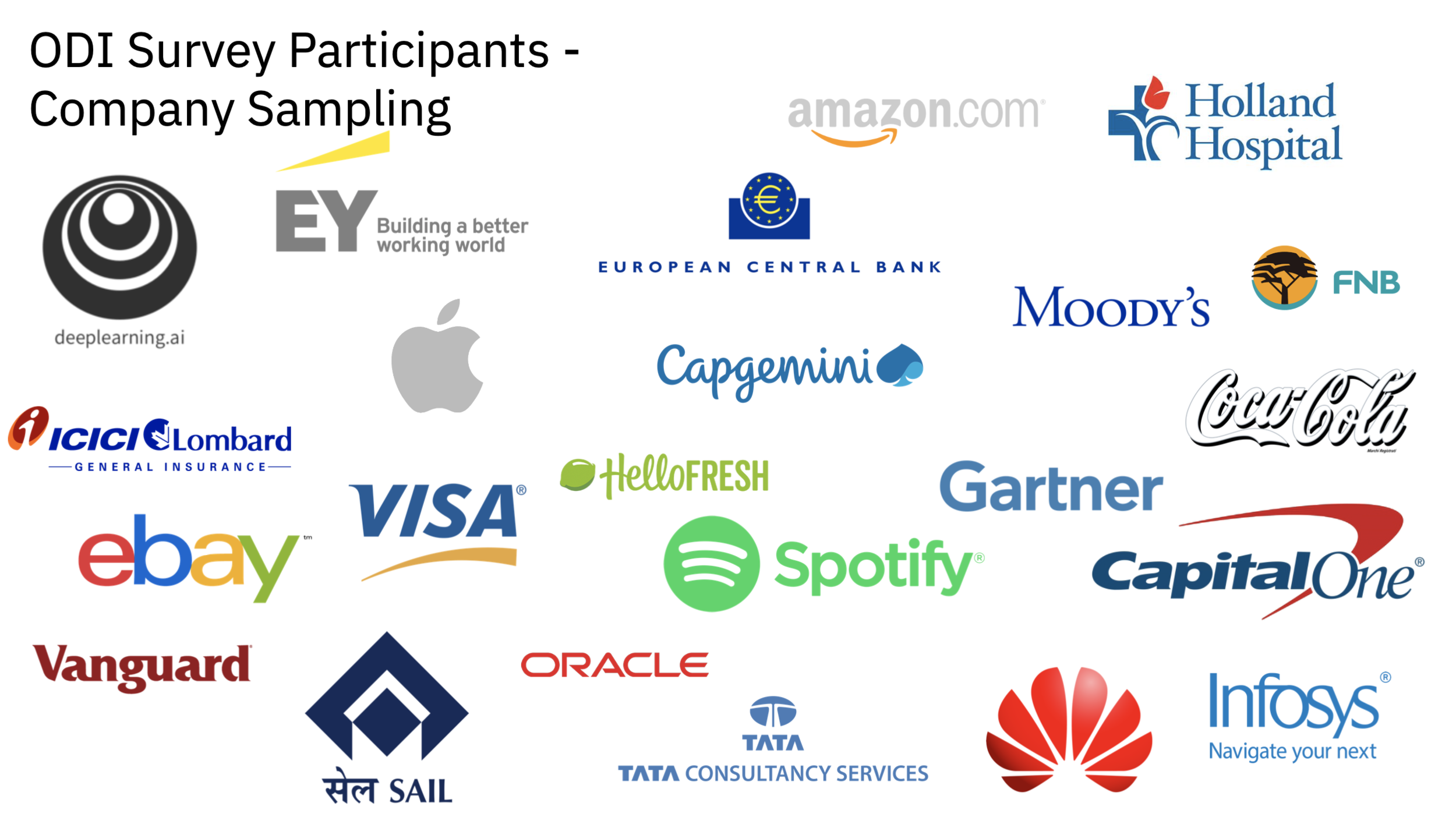

Sent survey to over 15k prospective and current customers sourced via Respondent, UserInterviews, LinkedIn, UX program, and Braze
Analyzed 161 qualified and completed responses as part of results
Ranked outcome statements according to opportunity scores


Outcome statements were prioritized and plotted based on participant ratings:
Table Stakes: Desired outcomes that are very important and very satisfied by existing products - a saturated market. To stay competitive while addressing these needs you will need to leave users extremely satisfied.
Underserved: Desired outcomes that are important and poorly satisfied - an unmet need. This is the biggest opportunity area.
Not Worth Going After: Desired outcomes low in importance and possibly already satisfied.
Reflection & Takeaways
Productivity Within Teams
Creating well structured teams and providing clear direction will help eliminate any slowdowns.
Divide up squads by user role (ex: data engineer, data steward, etc) and assign a squad lead to get things moving.
Keep teams small to speed up work & boost accountability. Smaller teams for smaller efforts vs large teams with too many people.
Create standing blocks of time on everyone's calendars for designated JTBD meetings or for cross-squad syncs.
Process Consistency
Following a standard process will help your team stay aligned and organized from end-to-end of your JTBD study.
Set a step-by-step approach that all teams will follow.
Establish standard structures/templates for each task, like interview protocols, synthesis boards (using mural), job maps, ODI, etc.
Ensure each member understands the desired end outcomes.
Look at examples: Seek out completed JTBD studies of other teams to know what to expect.
Organize Data
Ensuring that each member is aligned on tool use (such as Airtable) will help keep the Jobs to Be Done study organized.
Make sure every team is aligned on data input so it doesn't get out of hand. Establish clear editing responsibilities and limit the "creator" role if necessary.
Assume that everyone is a newbie to the tool; don't assume that everyone knows how to use it. Review the tool setup as a team and how to do certain functions.
Ensure that members know how to use the tool for cross-squad visibility purposes.